Testing AI fairness in predicting college dropout rate
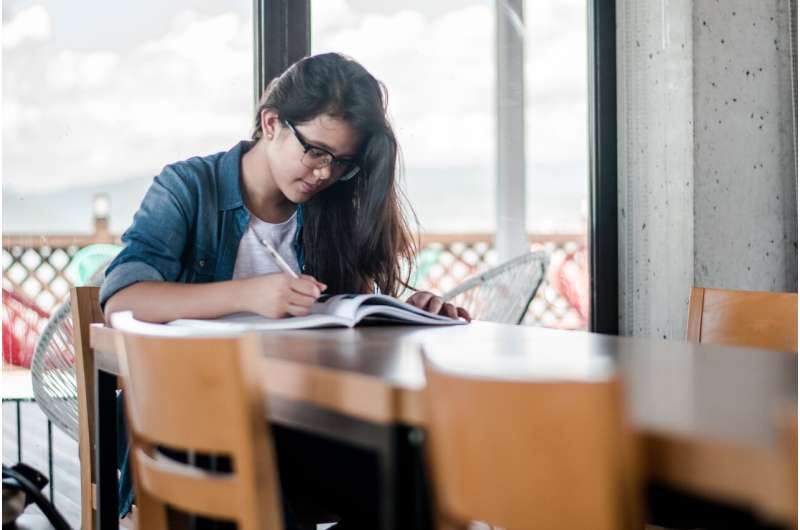
To enable having difficulties university college students in advance of it is far too late, much more and extra universities are adopting machine-understanding products to determine college students at threat of dropping out.
What information goes into these styles can have a massive impact on how precise and reasonable they are, specifically when it will come to shielded university student features like gender, race and loved ones cash flow. But in a new study, the greatest audit of a school AI process to day, researchers locate no proof that eliminating guarded student qualities from a model increases the accuracy or fairness of predictions.
This consequence came as a surprise to René Kizilcec, assistant professor of information science and director of the Future of Finding out Lab.
“We envisioned that removing socio-demographic qualities would make the design much less precise, since of how established these characteristics are in researching academic accomplishment,” he explained. “Despite the fact that we uncover that including these characteristics gives no empirical advantage, we endorse such as them in the product, since it at the extremely minimum acknowledges the existence of academic inequities that are even now linked with them.”
Kizilcec is senior writer of “Need to College Dropout Prediction Versions Include things like Guarded Characteristics?” to be presented at the digital Affiliation for Computing Equipment Meeting on Learning at Scale, June 22-25. The work has been nominated for a conference Greatest Paper award.
Co-authors are Long term of Understanding Lab members Hannah Lee, a master’s scholar in the area of computer system science, and lead author Renzhe Yu, a doctoral scholar at the College of California, Irvine.
For this function, Kizilcec and his workforce examined info on students in equally a residential college location and a totally on line software. The institution in the research is a big southwestern U.S. general public university, which is not named in the paper.
By systematically comparing predictive products with and devoid of protected characteristics, the scientists aimed to determine each how the inclusion of secured characteristics impacts the precision of higher education dropout prediction, and regardless of whether the inclusion of guarded attributes has an effect on the fairness of college dropout prediction.
The researchers’ dataset was huge: a overall of 564,104 residential training course- having data for 93,457 exclusive learners and 2,877 exclusive programs and 81,858 on line training course-having information for 24,198 exclusive learners and 874 unique programs.
From the dataset, Kizilcec’s team built 58 identifying options across four types, such as four shielded attributes—student gender first-technology faculty standing member of an underrepresented minority team (described as neither Asian nor white) and large financial want. To establish the implications of making use of shielded attributes to forecast dropout, the scientists produced two aspect sets—one with protected characteristics and one particular devoid of.
Their principal obtaining: Which include four significant safeguarded characteristics does not have any important influence on 3 widespread steps of overall prediction performance when commonly made use of attributes, such as academic data, are by now in the product.
“What issues for identifying at-threat learners is currently described by other attributes,” Kizilcec mentioned. “Secured attributes don’t increase a lot. There may well be a gender gap or a racial hole, but its association with dropout is negligible in comparison to characteristics like prior GPA.”
That mentioned, Kizilcec and his workforce still advocate for which include secured characteristics in prediction modeling. They note that greater education knowledge reflects longstanding inequities, and they cite new do the job in the broader device-understanding local community that supports the notion of “fairness by way of consciousness.”
“There is certainly been do the job displaying that the way specific attributes, like academic document, affect a student’s chance of persisting in college or university may possibly change across diverse safeguarded-attribute groups,” he explained. “And so by including scholar features in the design, we can account for this variation across distinctive pupil teams.”
The authors concluded by stating: “We hope that this review conjures up additional scientists in the discovering analytics and academic information mining communities to engage with troubles of algorithmic bias and fairness in the versions and devices they establish and consider.”
Kizilcec’s lab has carried out a good deal of get the job done on algorithmic fairness in schooling, which he said is an understudied topic.
“That is partly since the algorithms [in education] are not as visible, and they often do the job in different strategies as in comparison with felony justice or medication,” he mentioned. “In instruction, it can be not about sending a person to jail, or currently being falsely diagnosed for cancer. But for the unique university student, it can be a massive offer to get flagged as at-chance.”
New equipment studying design could get rid of bias from social network connections
Renzhe Yu et al, Should Higher education Dropout Prediction Versions Consist of Secured Characteristics?, Proceedings of the Eighth ACM Conference on Discovering @ Scale (2021). DOI: 10.1145/3430895.3460139
Cornell College
Quotation:
Tests AI fairness in predicting higher education dropout rate (2021, June 17)
retrieved 18 June 2021
from https://phys.org/news/2021-06-ai-fairness-higher education-dropout.html
This doc is topic to copyright. Apart from any good working for the purpose of private review or analysis, no
part may be reproduced without having the published permission. The written content is offered for information reasons only.